August 2, 2024
More insight and personalization: Lexer predictive product analytics
Introducing improved AI-powered predictive analytics and product recommendations in Lexer.
We are excited to share a major enhancement to our product recommendations, delivering more relevant, timely, and useful suggestions tailored to your needs. This update will improve product insight, drive greater 1:1 personalization, and enhance all marketing use cases.
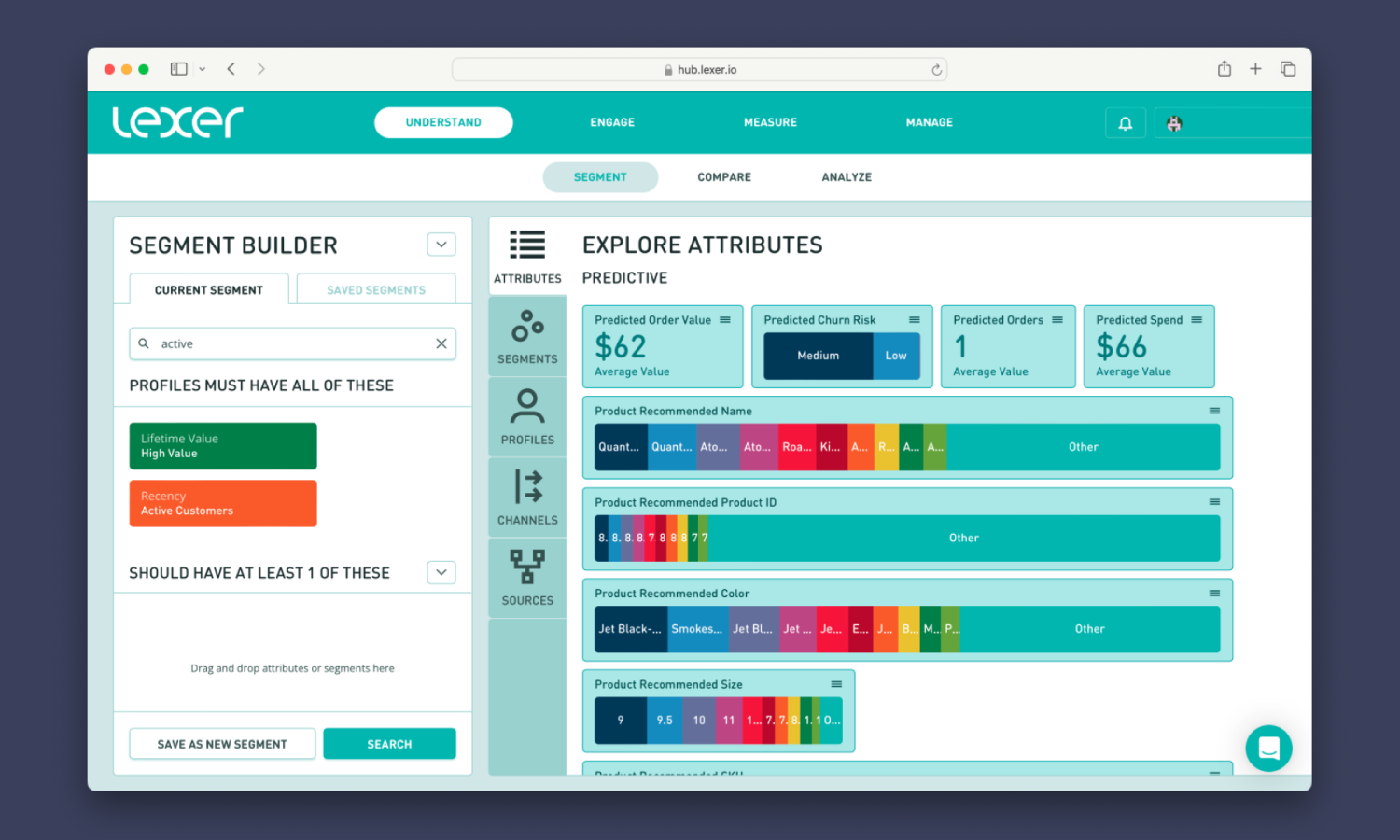
Why predictive analytics matter
Predictive analytics, specifically product recommendations, are more than just a nice to have—they’re a vital component of a robust customer-centric CDXP strategy. Here’s why:
Greater personalization. Recommendations harness transactional and behavioral data to offer customers a more personalized shopping experience. This tailored approach significantly boosts customer engagement and satisfaction. Recommendations feature heavily in our 1:1 AI messaging product, Contact, these messages will now be even more relevant.
Revenue growth. By suggesting products that resonate with customers' past behavior and preferences, recommendations increase future average order value (AOV) and drive repeat purchase (LTV). They serve as a powerful tool for upselling and cross-selling.
Increased customer retention. Offering relevant product suggestions enhances customer satisfaction, fostering loyalty and encouraging repeat visits. Happy customers are more likely to return, reducing churn and building long-term relationships.
New actionable insight. Analyzing recommendation patterns helps identify emerging trends and customer preferences, providing valuable insights to refine your marketing strategies, campaigns, merchandising, and product offerings.
In the below Lexer CDXP customer profile example, we can see that Aubrey is a low churn risk. She is likely to be interested in Eco Friendly, Performance Fabrics, Tees and Tops products. And is likely to buy dark colors next based on her previous purchase history.
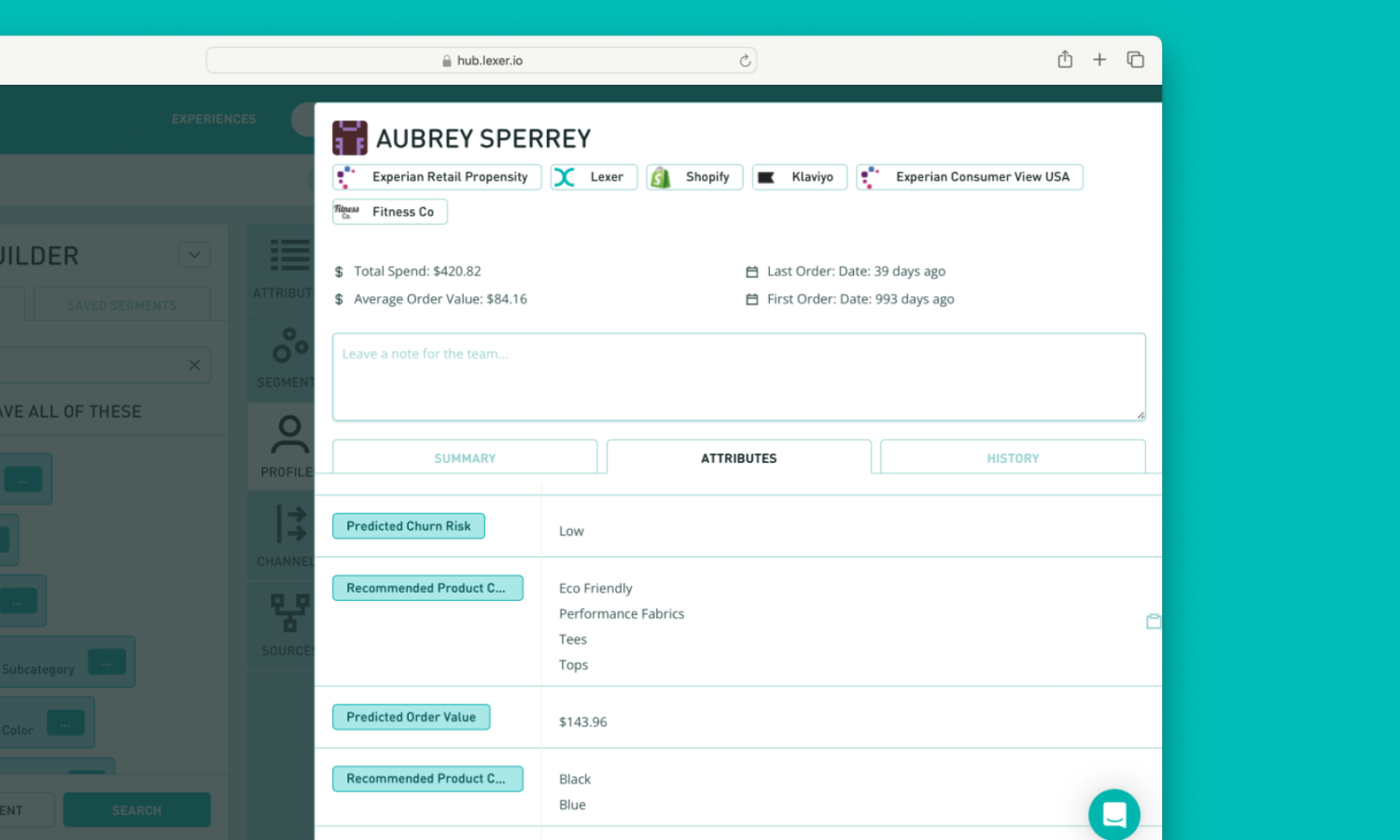
Improving recommendations
Our recommender uses machine-learning (AI) to take a customer’s transaction history, purchase date, product purchased, product id, and transaction amount, to predict their future purchasing behavior. Then recommends products that other customers with similar behaviors have purchased.
The approach to further improving recommendation logic focused on these five key areas. Our goal, to improve the quality of the results, and provide customer-centric retailers with more advanced analytics, and more control.
- Remove unsuitable items. Automatically remove unsuitable items from results, such as shipping, promotional products, free samples, bundles, and low price items.
- Inventory aware. Only recommend products that are in-stock, in-season, and available, ensuring availability and relevance.
- Boost recency. Recent customer shopping behavior has a stronger influence on the recommendations, making them more timely and topical.
- Increase coverage. Make sure as many CDXP customer profiles as possible receive recommendation values.
- Provide more control. Provide clients with the tools to fine-tune based on their needs, improving results, and improving user experience.
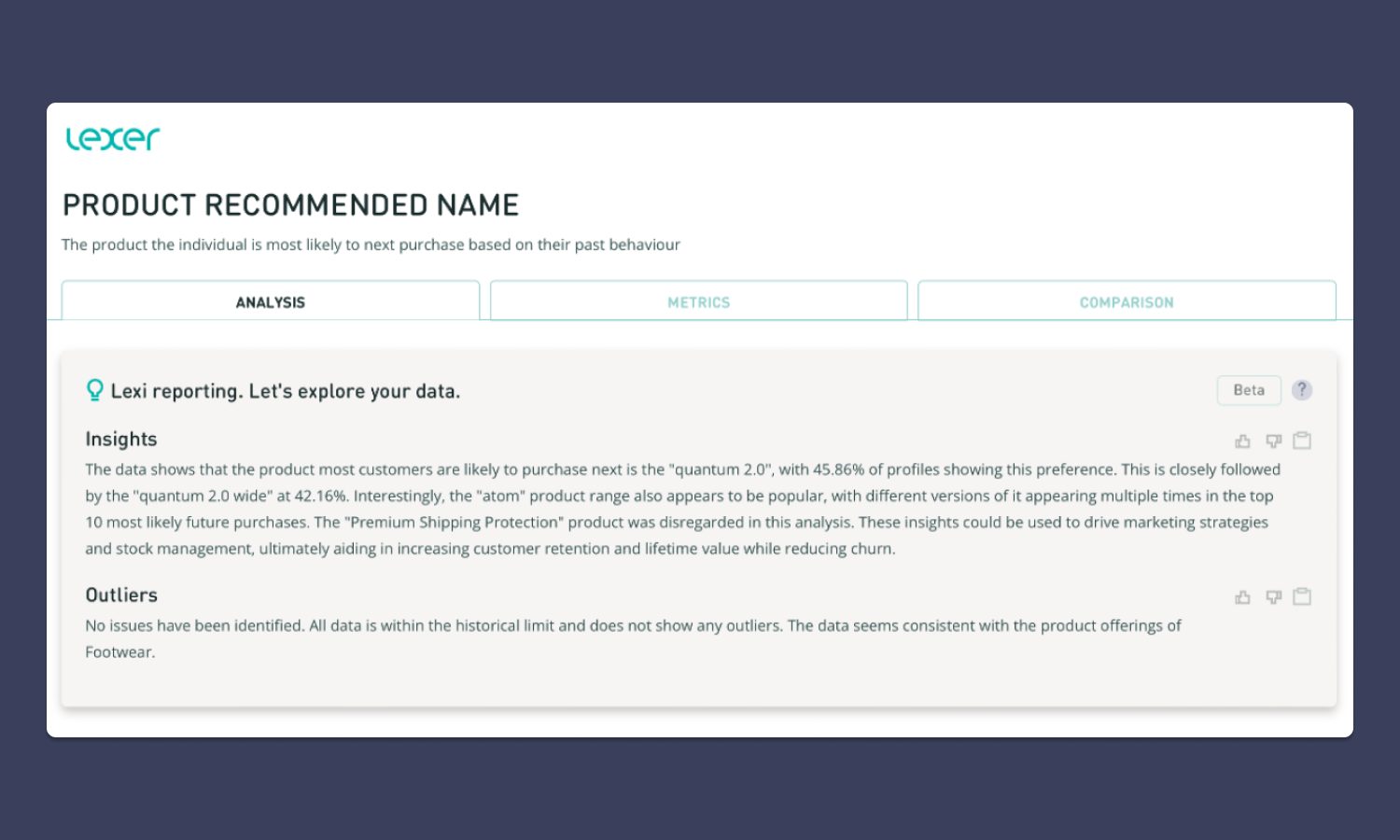
Data science made easy
We’ve introduced eight new adjustable settings (parameters) to help fine-tune and customize your recommendations even further. These easy to use settings provide all business users the type of control that was previously only available to data scientists and analysts.
- Recommendations per profile - choose how many recommendations are generated for each customer profile.
- Product price - set a minimum price, this will help exclude free items, samples, or low-value items you’d rather not recommend.
- Product name - exclude specific product types, names, or SKUs.
- Recency - set a time since the product was last sold, used to exclude out of season, older, or less frequently purchased products.
- Status - exclude products based on their Shopify status. Status options include; drafted, archived, or active.
- Previously purchased - choose if you want the recommendations to include products the customer has purchased before.
- Stock level - exclude based on Shopify low inventory levels, or out-of-stock items.
- Returns - exclude returned products from future recommendations.
Learn more about Lexer's full suite of predictive attributes, including predicted spend, predicted orders, and churn risk here.
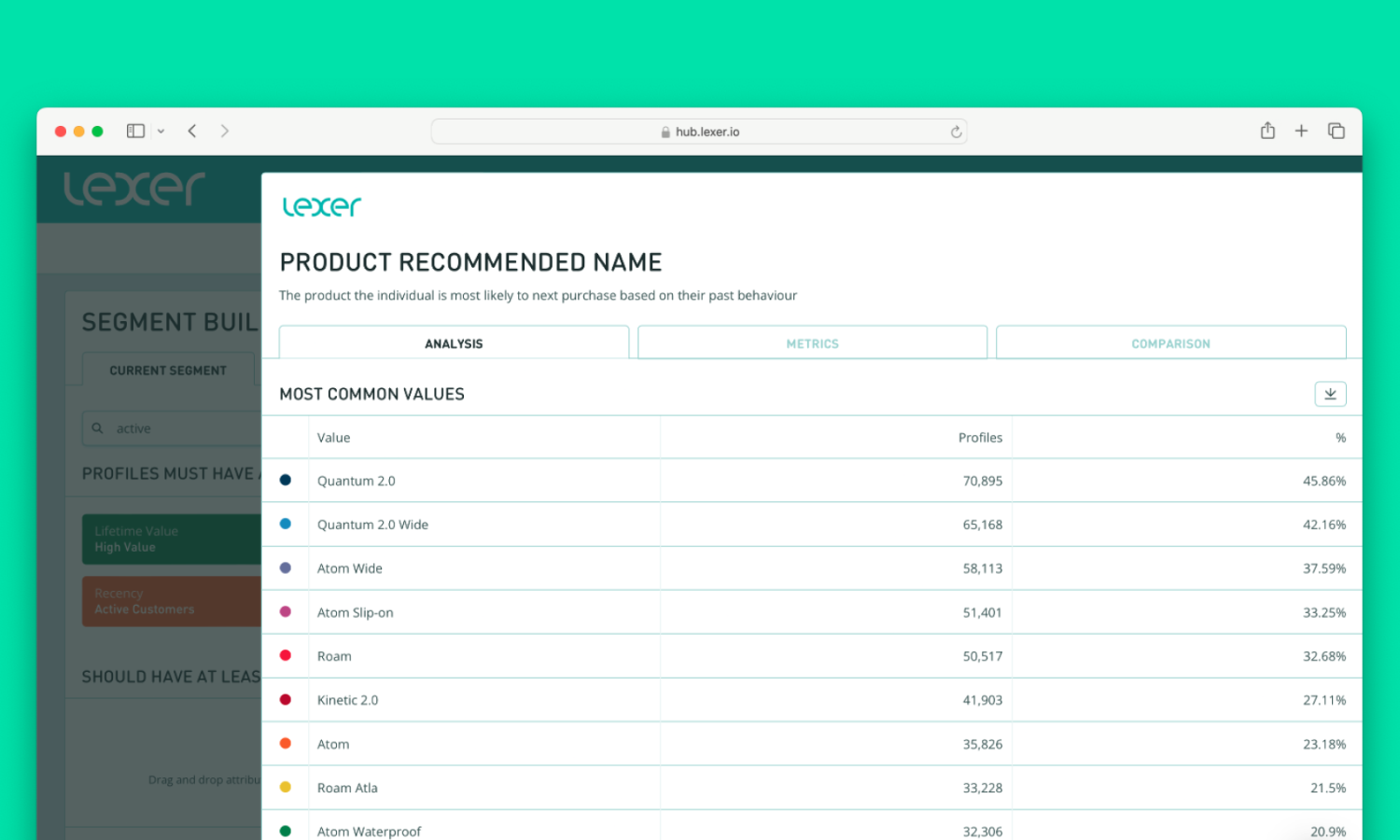
More relevancy, variety, and coverage
The new and improved AI-powered predictive analytics product recommendation logic is now available, and has automatically been deployed to Lexer CDXP client hubs.
- We’ve seen the number of customer profiles with a product recommendation increase by +16%. Recommended product name attribute coverage increased from 86% to 100%.
- By removing less relevant, and low-value items, the number of unique products being recommended increased by 53%, providing more variety, and personalization opportunities.
- Zero out of stock items appearing in recommendations.
- And finally, on average the number of product recommendations per customer profile increased by 50%, from four, to now six per profile. Helping to provide more product insight and provide more options for 1:1 personalization use cases.
Stay tuned for more updates as our customer-first predictive analytics continue to evolve. What’s next? We are looking to add more inputs to the recommendation calculations, such as marketing, and web behaviors, and offline signals too, powered by our in-store clienteling tool, Serve.
Interested in learning more about how Lexer can help you improve your customer analytics, engagement, and grow sales? Book a demo with one of our retail experts today.
Speak with our retail experts
